AI Geographic Profiles
Xorre’s AI technology, at its core, leverages real-world data to generate captivating narratives, with geographic profiles serving as the cornerstone of this process to ensure content relevance to a broad audience. This innovative approach facilitates the creation of content that resonates deeply with users, taking into consideration their specific locations and corresponding cultural nuances.
The same advanced technology drives profile creation for both entertainment and security applications, utilizing identical data sets for a harmonized and comprehensive approach. Whether it’s for creating immersive entertainment experiences or fortifying security systems, Xorre’s AI ingeniously uses geographic profiling to deliver solutions that are both engaging and effective.
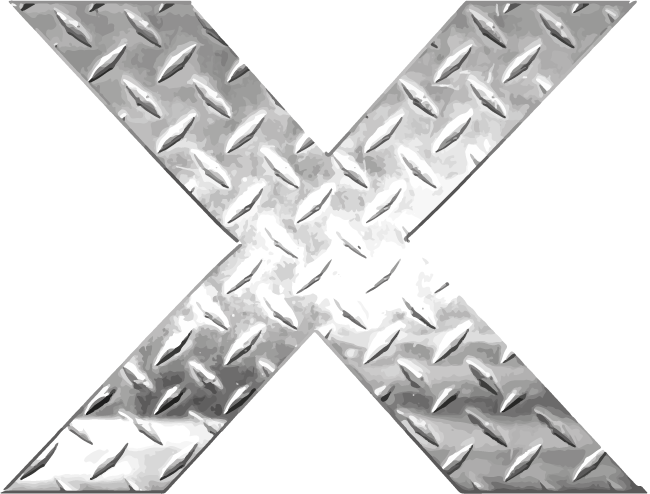
Reverse Network Place Analysis
Xorre’s AI employs a sophisticated technique known as Reverse Network Place Analysis, aimed at neutralizing potential adversaries’ use of Machine Learning (ML) by generating a deceptive dataset. This approach is specifically designed to counteract the application of geographical analysis tools.
The core idea is to leverage the capabilities of ML and AI to reverse-engineer a dataset that will lead to a predetermined conclusion when an adversary applies ML to it. In essence, Xorre’s AI is programmed to produce the “answer” you want it to give, effectively fabricating digital evidence to meet the desired outcome. This innovative method offers a powerful tool for manipulating data in a way that safeguards crucial information while confounding potential threats.
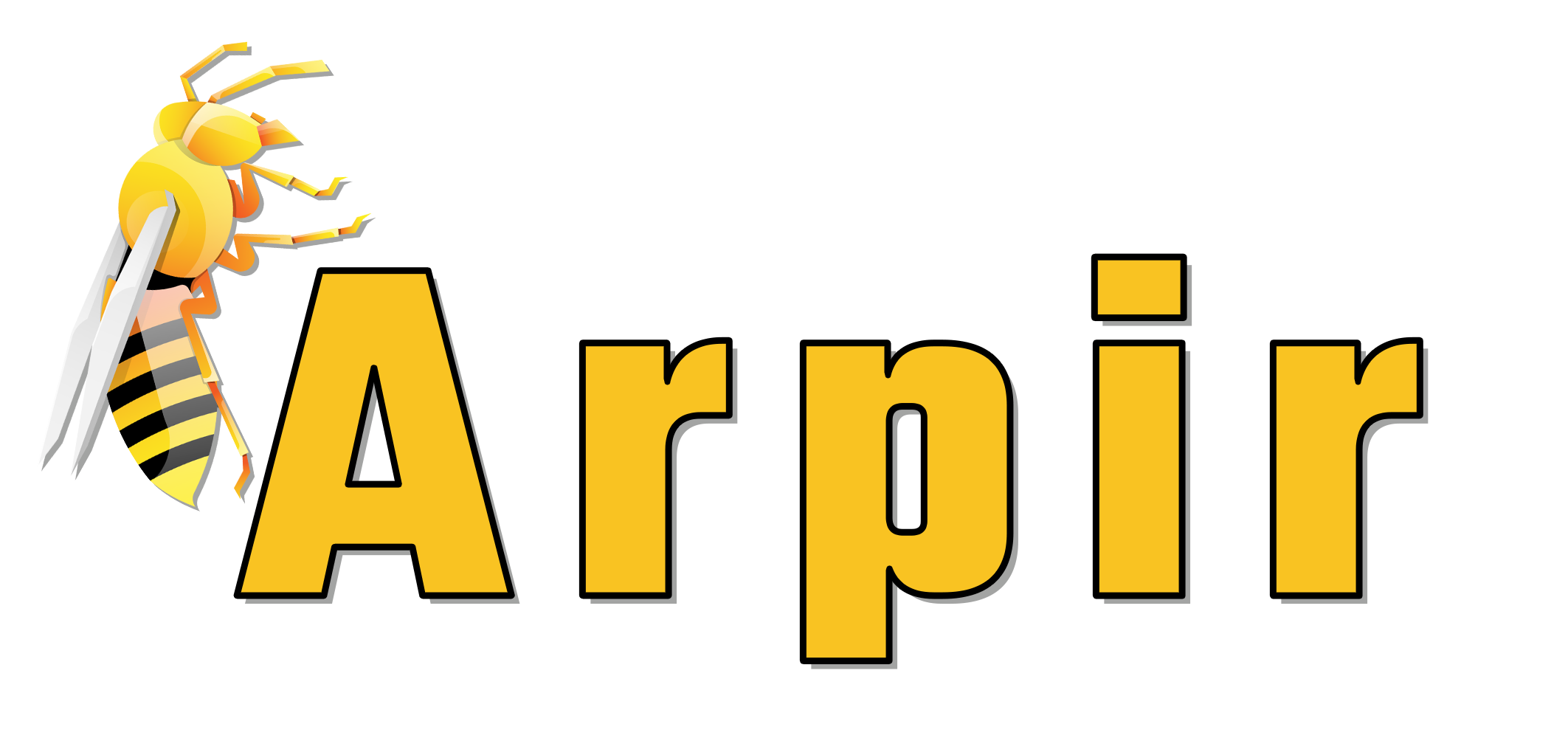
Now in Beta
See how AI creates Geographic Profiles:
Water
The first step in the process is to use maps of water to map out the boundaries of the land. This allows us to take into account proximity to shore as a crucial factor in our creation engine. Depending on the context, we can either desire proximity to shore or avoid it.
Off Shore Locations
Our procedural generation engine is also able to generate offshore locations, and uses a variety of factors, including proximity to land-based points of interest (POIs) such as ports and cities.
When generating offshore locations, the engine prioritizes those that are close to land-based POIs for 80% of the generated locations. However, for the remaining 20%, it prioritizes the most remote locations, such as uninhabited islands, for a more diverse range of offshore locations.
Roads
The next step in our procedural generation engine is building a detailed model of the roads in the area. We track major and minor roads separately, using machine learning algorithms to analyze the data and generate a realistic network of roads that accurately reflects the real world. This includes taking into account factors such as traffic flow and metro routes to identify relevant locations.
Activity Density
In addition to the roads themselves, we also take into account activity density in the area. This includes tracking data on population density, economic activity, and even crime rates. This information is used to generate points of interest (POIs) that are close to activity centers, such as busy commercial districts or high-traffic areas.
Land Use
The next step in our procedural generation engine is analyzing land use by using algorithms to analyze data on the types of buildings and structures in a given area, as well as their usage.
For example, we might be interested in generating POIs such as parks, retail areas, restaurants, or other gathering spots. We could also be looking for more remote or industrial areas, or locations around security services such as police stations or fire departments.
API Integration
Whatever the end goal, we are able to use information from a wide range of APIs and datasets to create real-time and evolving models that are infinitely scalable.
Elevation
Elevation data is an essential component of our procedural generation engine, and we use it to create highly detailed and realistic landscapes.
We have a variety of sources for this information, including satellite data, lidar data, and even ground surveys. The ease of obtaining and using this data is one of the key strengths of our engine. With modern technology, it is easy to get a hold of high-resolution elevation data from various sources, and our engine is able to process and optimize this data with minimal effort.
Smooth Outputs
Our engine uses advanced algorithms to optimize the elevation data, ensuring that it is accurate, detailed, and efficient. This includes processes such as smoothing out jagged terrain, filling in gaps in the data, and even removing unnecessary details.
Population Density
Population density maps are a key component of our procedural generation engine, and we use them to create POIs that are located around the most people. This is done by analyzing population density data and identifying the densest areas in a given region.
Once these areas have been identified, we then look at the housing in the area, such as apartments and houses, as well as other factors such as schools, hospitals, and parks. By doing this, we can generate POIs that are located in the most densely populated areas, ensuring that the generated landscapes are both realistic and functional.
Retail and Commerce Points
Another important aspect of our engine is identifying major retail and commerce centers. These areas are typically located in the densest areas, and are characterized by a high concentration of shops, restaurants, and other businesses. By identifying these areas, we can use them as starting points to generate additional POIs, such as supermarkets, gas stations, and other essential services.
Seasonal Events
Our procedural generation engine is designed to intelligently monitor and incorporate seasonal events into the Point of Interest (POI) creation process. This dynamic system can generate contingent profiles based on areas active during specific times of the year, like local holidays, festivals, sporting events, or rallies.
Leveraging a variety of data sources, the engine monitors calendars and schedules in real-time, ensuring dynamic and contextually relevant POI creation.
For example, if there are areas that are especially active during particular times of the year, such as local holidays, festivals, sporting events, or rallies, we can create these contingent profiles.
The engine is able to monitor calendars and schedules in real-time using a variety of data sources, and can create POIs dynamically.
Metro and Transit
Our procedural generation engine also uses local metro and transit schedules to create time-based POIs.
To do this, we start with the population centers identified earlier, and then create paths between them and other POIs we identified. We then look at metro and transit schedules to identify routes and times along the transit lines, and use this information to create POIs that correspond with these.
For example, if a metro line runs through a particular area, we can create POIs such as stations, ticket vending machines, and other features that correspond with the route and schedule of the metro. We can also create POIs that reflect the activity along the transit line, such as shops, restaurants, and other businesses that are located near metro stations. By doing this, we can create models that reflect the daily rhythms and patterns of the real world.